How to Have AI-Ready Data
- Co-Author: Kelly Grosskreutz - Modern Work Practice Manager
- Co-Author: Josh Marek - Data and AI Solutions Manager
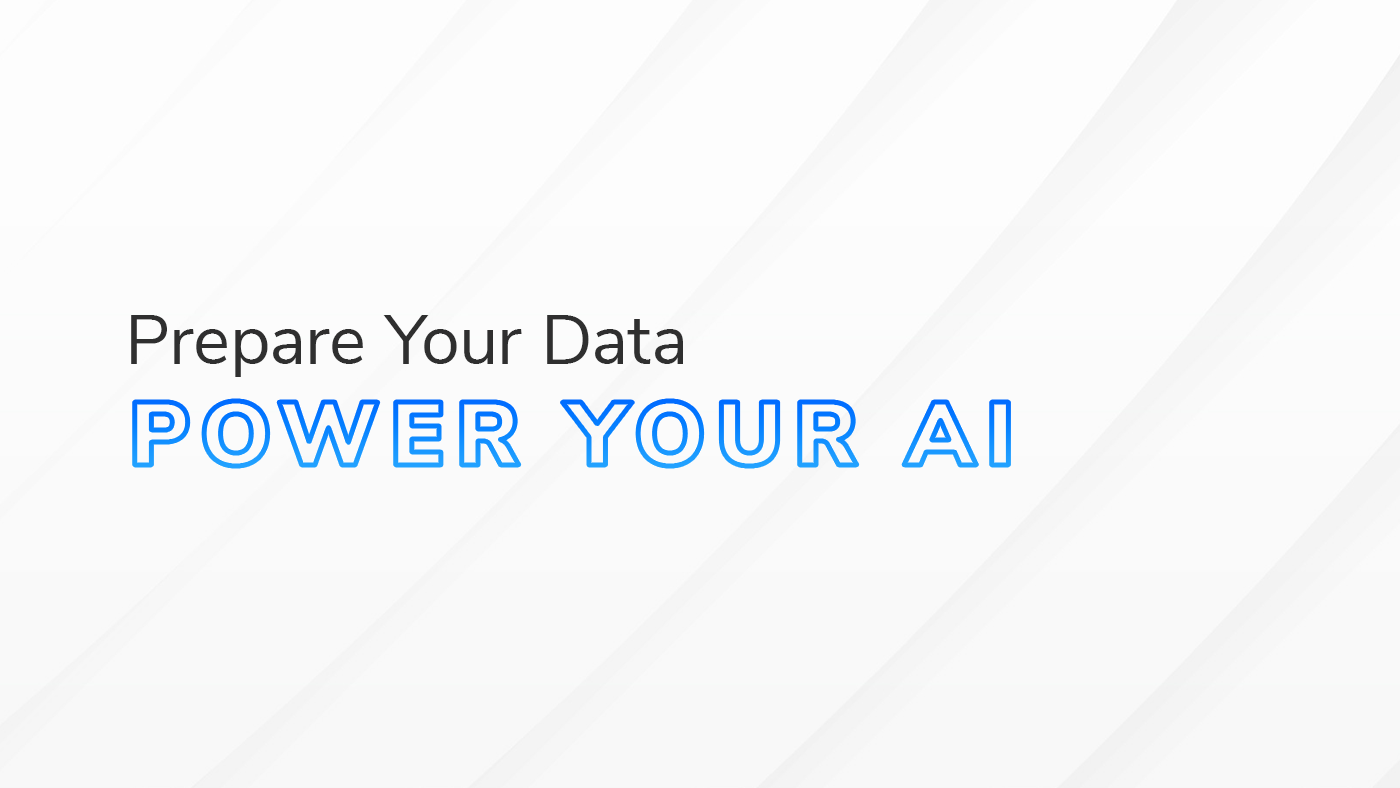
Artificial intelligence (AI) is only as good as the data it processes.
AI tools, both internal organizational and commercial systems, sift through almost incomprehensible amounts of data daily—parsing, categorizing, refining, repeating—while completing their intended tasks.
For organizations aiming to leverage AI, ensuring good data hygiene is one of the most crucial steps in producing desired outcomes.
Clean, well-prepared data leads to accurate, reliable insights. Our goal is to help provide effective data preparation practices you can implement now, setting your AI initiatives up for success.
Understanding Data Preparation
Data preparation involves collecting, cleaning, and transforming raw data into a format suitable for data analysis. This process lays the foundation for any successful AI/ML project.
Effective data preparation addresses issues like missing values, inconsistencies, and irrelevant information, making your data more reliable and easier for AI to analyze.
Preparing to Prepare: Key Considerations for AI-Ready Data
- Define Objectives: Clearly outline the goals of your AI project. Knowing what problem you're trying to solve guides the selection and preparation of your data, ensuring relevance and focus. Well-defined objectives also help measure your AI initiatives' success and impact.
- Data Quality Over Quantity: Prioritize high-quality data over simply amassing large volumes. Clean, accurate, and relevant data significantly improves system performance, providing more reliable and actionable insights. It’s usually better to have a smaller dataset of pristine data than a large dataset filled with noise and errors.
- Continuous Refinement: Understand that data preparation is an ongoing process. Regular updates and improvements are necessary as new data comes in and your AI system(s) evolves. Continuously refining your data ensures that your AI system remains accurate and effective over time.
- Incorporating AI Governance: Integrate AI Risk Management Framework (RMF) and AI Governance practices into your data preparation. These frameworks help you manage risks, maintain compliance, and ensure ethical AI use. They provide guidelines for data management, ensuring your AI systems are both robust and trustworthy.
A Periodic Audit will provide assurance that your data is the right data for your AI systems.
Steps to Effectively Prepare Your Data for AI
Step 1: Ensure Data Integrity and Compliance for AI Systems
Your top priority when preparing data for AI systems is to make certain that the data your AI models will use is precisely what you intend. This involves governance and implementing guardrails to prevent privacy breaches and address any possible ethical issues.
Identify and classify your data sources. Map where each piece of data originates, whether from internal systems like CRM databases or external sources like public websites. Categorize data based on sensitivity and compliance requirements—like public, internal, confidential, and restricted.
Implement strict data access controls to ensure your AI systems use only the intended data. Use role-based access control (RBAC) to restrict access based on user roles, encrypt sensitive data, and maintain audit trails to track data access and modifications. Consider assigning data stewards to oversee data integrity and security, as well as adherence to data governance policies.
Step 2: Collect Data
Effective data preparation for machine learning starts with gathering both structured and unstructured data to establish a comprehensive and diverse dataset
- Structured Data: Data organized in a defined manner, such as databases and spreadsheets. Examples include customer databases, sales records, and financial data
- Unstructured Data: Data that lacks a predefined format, such as text documents, images, and videos. This will likely be the majority of your data—as much as 90% of all data is defined as unstructured.
It’s important to gather as much relevant information as possible to create a comprehensive dataset when collecting data. This diversity allows your AI model to learn from a wide range of scenarios and make more accurate predictions.
Step 3: Clean Data
Data cleaning involves identifying and rectifying errors, inconsistencies, and missing values. This step helps eliminate noise that can skew AI model results. The key activities in data cleaning are
- Removing Duplicates: Identifying and eliminating duplicate records to prevent distorted analysis. For instance, duplicate customer records can lead to inaccurate customer segmentation
- Correcting Errors: Fixing incorrect data entries, such as misspellings or inaccurate numerical values. For example, correcting "Nwe York" to "New York" allows for consistent geographical data.
- Handling Missing Values: Addressing gaps in the data, either by filling in missing values or removing incomplete records. Techniques like mean imputation or regression imputation can be used to estimate missing values—only employ these practices if it makes sense to do so
Clean data allows your AI system to learn from accurate and reliable information, leading to better performance.
Step 4: Transform Data
Data transformation involves converting data into a format that AI systems can easily understand and work with. Think of it as making the data more readable and organized for AI so that it can give you better results.
- Normalization: This is scaling numbers to a standard range, like 0 to 1. For example, if you have income and age data, normalization safeguards against one overpowering the other in the AI's learning process. It’s putting everything on the same scale so the AI can compare them fairly.
- Encoding Categorical Variables: Some data, like "Yes/No" or "High/Medium/Low," needs to be turned into numbers simply because AI works better with numbers. Techniques like one hot encoding or label encoding help convert these categories into a format the AI can use. Think of it as translating words into a language the AI understands.
- Aggregating Data: This involves combining several data points into one summary. Instead of looking at daily sales data, you can sum it up to get monthly totals. This makes the data easier to analyze and reduces the volume of data the AI needs to process.
Proper data transformation assures that your data is in a format that AI systems can effectively process, making it easier for the AI to learn from the data and make accurate predictions.
Step 5: Data Reduction
Data reduction simplifies your dataset by removing unnecessary or irrelevant information, keeping only what’s most important. This makes the AI's job easier and improves its performance.
- Feature Selection: Pick out the most relevant pieces of data for your AI model. Techniques like correlation analysis and recursive feature elimination help decide which features to keep
- Dimensionality Reduction: This reduces the number of data points while keeping the essential information intact. Methods like Principal Component Analysis (PCA) and t-Distributed Stochastic Neighbor Embedding (t-SNE) help achieve this. Think of it as summarizing a long story into its main points.
Focusing on the most important data makes your AI model more efficient and faster to run. This helps the AI learn better and gives quicker, more accurate results.
Optimize Your Data for AI
Your best AI results will come, not from a lot of data, but from the right kind of data. Properly optimizing your data will take your organization’s raw information and drive smarter decisions and better outcomes.
You need a structured, accessible, and well-organized data environment:
- O
ptimize Data Structure: AI works best with a solid organizational structure. For example, having clear definitions for departments and teams helps businesses extract more precise insights from AI tools. This allows AI to understand and predict interactions between different parts of the organization, leading to improved business processes.
- Organize Your Data Architecture: Make sure your data is well-organized and easy to access. This might mean setting up a centralized data storage system like a data lake, lakehouse, or warehouse. Organized data architecture makes it easier for AI to process and analyze information.
- Break Down Data Silos: Data often gets stuck in separate departments, making it hard for AI to get a complete picture. Encourage collaboration between departments and make sure all relevant data is accessible for AI analysis. Breaking down these silos helps AI to provide more comprehensive insights.
- Focus on Structure, Not Just Size: While having a lot of data can be useful, it's more important that the data is well-organized. Structured data allows AI to extract more accurate insights and make better predictions. A well-organized, smaller dataset is often more valuable than a large, disorganized one.
By optimizing your data, you're setting up your AI systems for success. Clean, structured data fuels your AI engine, leading to sharper insights and smarter business decisions.
Get a Head Start with AI-Ready Data
From defining clear objectives and prioritizing high-quality data to implementing strong governance and continuous refinement, each data preparation step you take brings you closer to optimizing your AI systems. Collecting diverse, relevant data and maintaining its integrity through cleaning and transformation processes will lead your AI systems to perform at their best.
Optimizing your data structure, breaking down silos, and organizing your data architecture not only enhance AI performance but also streamline your business processes. As you focus on these practices, you pave the way for AI to position your organization as a leader in innovation and efficiency.
These data preparation strategies allow you not only to prepare for AI, but also prepare for success. Your efforts today will drive the intelligent business decisions of tomorrow, giving you a competitive edge.
From helping you define your objectives and protecting data integrity to optimizing your data architecture and breaking down silos, HBS provides the expertise and support you need to set your AI initiatives up for success.
Contact HBS today so we can work together to transform your data into an integral business driver.